Stephen J. Verzi
Senior Member of Technical Staff
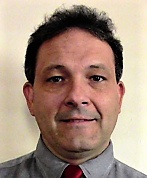
Biography
Education
- Ph.D., Computer Science, University of New Mexico – 2003
- Master of Science, Computer Science, University of New Mexico – 1990
- Bachelor of Science, Computer Science, December 1987
Publications
-
Naugle, A., Krofcheck, D., Warrender, C., Lakkaraju, K., Swiler, L., Verzi, S., Emery, B., Murdock, J., Bernard, M., Romero, V., & Romero, V. (2023). What can simulation test beds teach us about social science? Results of the ground truth program. Computational and Mathematical Organization Theory, 29(1), pp. 242-263. https://doi.org/10.1007/s10588-021-09349-6 Publication ID: 80604
-
Naugle, A., Verzi, S., Lakkaraju, K., Swiler, L., Warrender, C., Bernard, M., Romero, V., & Romero, V. (2023). Feedback density and causal complexity of simulation model structure. Journal of Simulation, 17(3), pp. 229-239. https://doi.org/10.1080/17477778.2021.1982653 Publication ID: 75723
-
Naugle, A., Rothganger, F., Verzi, S., Doyle, C., & Doyle, C. (2022). Conflicting Information and Compliance With COVID-19 Behavioral Recommendations. https://doi.org/10.2172/1894426 Publication ID: 80392
-
Smith, M., Carbajal, A., Domschot, E., Johnson, N., Goyal, A., Lamb, C., Lubars, J., Kegelmeyer, W., Krishnakumar, R., Quynn, S., Ramyaa, R., Verzi, S., Zhou, X., & Zhou, X. (2022). MalGen: Malware Generation with Specific Behaviors to Improve Machine Learning-based Detectors. https://doi.org/10.2172/1893244 Publication ID: 80379
-
Naugle, A., Swiler, L., Lakkaraju, K., Verzi, S., Warrender, C., Romero, V., & Romero, V. (2022). Graph-Based Similarity Metrics for Comparing Simulation Model Causal Structures. https://doi.org/10.2172/1884926 Publication ID: 80095
-
Naugle, A., Russell, A., Lakkaraju, K., Swiler, L., Verzi, S., Romero, V., & Romero, V. (2022). The Ground Truth Program: Simulations as Test Beds for Social Science Research Methods.. Computational and Mathematical Organization Theory, 29(1), pp. 1-16. https://doi.org/10.1007/s10588-021-09346-9 Publication ID: 80622
-
Verzi, S., Krishnakumar, R., Levin, D., Krofcheck, D., Williams, K., & Williams, K. (2022). Data Science and Machine Learning for Genome Security. https://doi.org/10.2172/1855003 Publication ID: 75932
-
Livesay, M., Pless, D., Verzi, S., Stamber, K., Lilje, A., & Lilje, A. (2022). A Theoretical Approach for Reliability Within Information Supply Chains with Cycles and Negations. IEEE Transactions on Reliability, 71(1), pp. 404-414. https://doi.org/10.1109/tr.2021.3068737 Publication ID: 77767
-
Verzi, S., Johnson, N., Khanna, K., Zhou, X., Quynn, S., Krishnakumar, R., Smith, M., & Smith, M. (2021). Malware Generation with Specific Behaviors to Improve Machine Learning-based Detectors [Conference Presenation]. https://doi.org/10.2172/1899508 Publication ID: 76852
-
Thorpe, J., Musuvathy, S., Verzi, S., Vugrin, E., Dykstra, M., Sahakian, M., & Sahakian, M. (2021). GAMVT: A Generative Algorithm for MultiVariate Timeseries Data [Conference Paper]. https://www.osti.gov/biblio/1886761 Publication ID: 75585
-
Yoon, H., Verzi, S., Cauthen, K., Musuvathy, S., Melander, D., Norland, K., Morales, A., Lee, J., Sun, A., & Sun, A. (2021). Predictive Data-driven Platform for Subsurface Energy Production. https://doi.org/10.2172/1821788 Publication ID: 75611
-
Matteo, E., Conley, D., Verzi, S., Roberts, B., Doyle, C., Sobolik, S., Gilletly, S., Bauer, S., Pyrak-Nolte, L., Reda Taha, M., Stormont, J., Crandall, D., Moriarty, D., John, E., Wilson, J., Bettin, G., Hogancamp, J., Fernandez, S., Anwar, I., … Bland, J. (2021). Advanced Detection of Wellbore Failure for Safe and Secure Utilization of Subsurface Infrastructure. https://doi.org/10.2172/1820250 Publication ID: 75660
-
Naugle, A., Doyle, C., Sweitzer, M., Rothganger, F., Verzi, S., Lakkaraju, K., Kittinger, R., Bernard, M., Chen, Y., Loyal, J., Mueen, A., & Mueen, A. (2021). Emergent Recursive Multiscale Interaction in Complex Systems. https://doi.org/10.2172/1821796 Publication ID: 75722
-
Johnson, N., Domschot, E., Khanna, K., Kegelmeyer, W., Lamb, C., Ramyaa, R., Smith, M., Verzi, S., Zhou, X., Carbajal, A., Haus, B., Ingram, J., & Ingram, J. (2021). Going Beyond Signature Malware Detection by Learning Behaviors [Conference Presenation]. https://doi.org/10.2172/1884420 Publication ID: 79195
-
Doyle, C., Gilletly, S., Verzi, S., Matteo, E., Roberts, B., Sobolik, S., Moriarty, D., & Moriarty, D. (2021). Integrating Machine Learning into a Methodology for Early Detection of Wellbore Failure [Conference Presenation]. https://doi.org/10.2172/1882311 Publication ID: 79257
-
Verzi, S., Guttromson, R., Sorensen, A., Jones, C., & Jones, C. (2021). AlphaGrid: Grid Stability Using Machine Learning State Space Navigation [Presentation]. https://www.osti.gov/biblio/1877788 Publication ID: 79260
-
Verzi, S., Krishnakumar, R., Levin, D., Krofcheck, D., Boskin, C., Williams, K., & Williams, K. (2021). Data Science for Characterization of Genome Noise/Mutation [Presentation]. https://www.osti.gov/biblio/1884061 Publication ID: 79291
-
Verzi, S., Krishnakumar, R., Levin, D., Krofcheck, D., Boskin, C., Williams, K., & Williams, K. (2021). Data Science for Detection of Genome Editing [Presentation]. https://www.osti.gov/biblio/1889012 Publication ID: 79387
-
Matteo, E., Roberts, B., Sobolik, S., Gilletly, S., Doyle, C., John, E., Verzi, S., & Verzi, S. (2021). Integrating Machine Learning into a Methodology for Early Detection of Wellbore Failure [Slides] [Conference Presenation]. https://doi.org/10.2172/1869382 Publication ID: 78527
-
Matteo, E., Roberts, B., Sobolik, S., Gilletly, S., Doyle, C., John, E., Verzi, S., & Verzi, S. (2021). Integrating Machine Learning into a Methodology for Early Detection of Wellbore Failure [Slides] [Conference Presenation]. https://doi.org/10.2172/1869384 Publication ID: 78530
-
Sahakian, M., Musuvathy, S., Thorpe, J., Verzi, S., Vugrin, E., Dykstra, M., & Dykstra, M. (2021). Threat Data Generation for Space Systems [Conference Presenation]. https://doi.org/10.2172/1873278 Publication ID: 78832
-
Sahakian, M., Musuvathy, S., Thorpe, J., Verzi, S., Vugrin, E., Dykstra, M., & Dykstra, M. (2021). Threat Data Generation for Space Systems [Conference Paper]. https://doi.org/10.1109/SCC49971.2021.00018 Publication ID: 78837
-
Yoon, H., Melander, D., Verzi, S., & Verzi, S. (2021). Machine Learning Application for Permeability Estimation of Digital Rock Images [Conference Presenation]. https://doi.org/10.2172/1859688 Publication ID: 77750
-
Yoon, H., Melander, D., Verzi, S., & Verzi, S. (2021). Machine learning application for permeability estimation of three-dimensional rock images [Conference Poster]. CEUR Workshop Proceedings. https://doi.org/10.2172/1833497 Publication ID: 71969
-
Rothganger, F., Naugle, A., Verzi, S., & Verzi, S. (2021). Behavioral Feedback in Disease Dynamics [Presentation]. https://www.osti.gov/biblio/1843095 Publication ID: 75171
-
Smith, M., Johnson, N., Ingram, J., Carbajal, A., Haus, B.I., Domschot, E., Ramyaa, R., Lamb, C., Verzi, S., Kegelmeyer, W., & Kegelmeyer, W. (2020). Mind the Gap: On Bridging the Semantic Gap between Machine Learning and Malware Analysis [Conference Presenation]. AISec 2020 – Proceedings of the 13th ACM Workshop on Artificial Intelligence and Security. https://doi.org/10.2172/1831009 Publication ID: 71665
-
Sahakian, M., Thorpe, J., Verzi, S., Musuvathy, S., Vugrin, E., Dykstra, M., Umbenhower, M., & Umbenhower, M. (2020). AIRSS: Adaptive Intrusion Response for Space Systems [Presentation]. https://www.osti.gov/biblio/1830982 Publication ID: 71304
-
Smith, M., Johnson, N., Ingram, J., Carbajal, A., Haus, B.I., Domschot, E., Ramyaa, R., Lamb, C., Verzi, S., Kegelmeyer, W., & Kegelmeyer, W. (2020). Mind the Gap: On Bridging the Semantic Gap between Machine Learning and Malware Analysis [Conference Poster]. https://doi.org/10.1145/3411508.3421373 Publication ID: 73995
-
Thorpe, J., Sahakian, M., Musuvathy, S., Verzi, S., Dykstra, M., Vugrin, E., & Vugrin, E. (2020). Threat Data Generation for a More Resilient Response [Conference Poster]. https://www.osti.gov/biblio/1811432 Publication ID: 74238
-
Sweitzer, M., Kittinger, R., Doyle, C., Naugle, A., Lakkaraju, K., Rothganger, F., Verzi, S., & Verzi, S. (2020). Communicative Vibration: A Graph-Theoretic Approach to Group Stability in an Online Social Network [Conference Poster]. https://www.osti.gov/biblio/1811443 Publication ID: 74242
-
Naugle, A., Rothganger, F., Verzi, S., & Verzi, S. (2020). Conflicting Information and Compliance With COVID-19 Stay-At-Home Orders [Conference Poster]. https://www.osti.gov/biblio/1811614 Publication ID: 74254
-
Smith, M., Carbajal, A., Domschot, E., Haus, B.I., Ingram, J., Johnson, N., Kegelmeyer, W., Lamb, C., Ramyaa, R., Verzi, S., & Verzi, S. (2020). MalGen: On Bridging the Semantic Gap between Machine Learning and Malware Analysis [Conference Poster]. https://www.osti.gov/biblio/1811972 Publication ID: 74314
-
Smith, M., Johnson, N., Ingram, J., Carbajal, A., Ramyaa, R., Domschot, E., Lamb, C., Verzi, S., Kegelmeyer, W., & Kegelmeyer, W. (2020). Mind the Gap: On Bridging the Semantic Gap between Machine Learning and Information Security [Conference Poster]. https://www.osti.gov/biblio/1782493 Publication ID: 73420
-
Yoon, H., Melander, D., Verzi, S., & Verzi, S. (2020). Permeability Prediction of Porous Media using Convolutional Neural Networks with Physical Properties [Conference Poster]. https://www.osti.gov/biblio/1773030 Publication ID: 73146
-
Verzi, S. (2020). Machine Learning at Sandia [Presentation]. https://www.osti.gov/biblio/1768146 Publication ID: 72778
-
Yoon, H., Melander, D., Verzi, S., & Verzi, S. (2020). Permeability prediction of porous media using convolutional neural networks with physical properties [Conference Poster]. CEUR Workshop Proceedings. https://www.scopus.com/inward/record.uri?partnerID=HzOxMe3b&scp=85083343043&origin=inward Publication ID: 66664
-
Guttromson, R., Sorensen, A., Jones, C., Verzi, S., Byrne, R.H., Hanley, C., & Hanley, C. (2019). AlphaGrid: Grid Stability Using Machine Learning State Space Navigation [Presentation]. https://www.osti.gov/biblio/1645709 Publication ID: 70283
-
Krishnakumar, R., Boskin, C., Levin, D., Carmody, D., Finley, P., Verzi, S., Williams, K., Carney, J., & Carney, J. (2019). Data Science for Detection of Genome Editi [Presentation]. https://www.osti.gov/biblio/1645734 Publication ID: 70337
-
Naugle, A., Lakkaraju, K., Balanaga, V., Warrender, C., Verzi, S., Livesay, M., Swiler, L., & Swiler, L. (2019). A Complexity-Focused Framework for Comparing and Evaluating Social Simulations [Presentation]. https://www.osti.gov/biblio/1645756 Publication ID: 70428
-
Guttromson, R., Verzi, S., Jones, C., Sorensen, A., Byrne, R.H., Hanley, C., & Hanley, C. (2019). Grid Stability Using Machine Learning State Space Navigation [Presentation]. https://www.osti.gov/biblio/1807097 Publication ID: 69670
-
Guttromson, R., Verzi, S., Jones, C., Sorensen, A., Byrne, R.H., Hanley, C., & Hanley, C. (2019). Grid Stability Using Machine Learning State Space Navigation [Presentation]. https://www.osti.gov/biblio/1645566 Publication ID: 69671
-
Naugle, A., Swiler, L., Lakkaraju, K., Verzi, S., Warrender, C., Bernard, M., Romero, V., & Romero, V. (2019). Graph-Based Similarity Metrics for Comparing Simulations and Causal Loop Diagrams [Conference Poster]. https://www.osti.gov/biblio/1641241 Publication ID: 69911
-
Hill, A.J., Donaldson, J., Rothganger, F., Vineyard, C., Follett, D., Follett, P., Smith, M., Verzi, S., Severa, W., Wang, F., Aimone, J.B., Naegle, J., James, C., & James, C. (2019). Neuromorphic Computing Algorithms and Architecture Research at Sandia [Presentation]. https://www.osti.gov/biblio/1645676 Publication ID: 70107
-
Lakkaraju, K., Naugle, A., Verzi, S., Swiler, L., Livesay, M., Warrender, C., Bernard, M., Romero, V., & Romero, V. (2019). Complexity metrics for Agent Based Models of Social Systems [Conference Poster]. https://www.osti.gov/biblio/1639730 Publication ID: 68092
-
Xu, G., Wang, Y., Verzi, S., & Verzi, S. (2019). Neural Networks for microscopic image analysis [Conference Poster]. https://www.osti.gov/biblio/1639485 Publication ID: 67621
-
Verzi, S. (2019). Layered Approach Builds Confidence in Complex Social Systems Modeling [Presentation]. https://www.osti.gov/biblio/1643885 Publication ID: 67055
-
Galiardi, M.A., Verzi, S., Birch, G., Stubbs, J., Woo, B.L., Kouhestani, C., & Kouhestani, C. (2018). Physical Security Assessment Using Temporal Machine Learning [Conference Poster]. Proceedings – International Carnahan Conference on Security Technology. https://doi.org/10.1109/CCST.2018.8585705 Publication ID: 63561
-
Xu, G., Wang, Y., Verzi, S., & Verzi, S. (2018). Machine learning assisted microscopic image analysis for unconventional reservoir studies [Conference Poster]. https://www.osti.gov/biblio/1761155 Publication ID: 60518
-
Dawson, L., Verzi, S., Levin, D., Melander, D., Sorensen, A., Cauthen, K., Wilches-Bernal, F., Berg, T., Lavrova, O., Guttromson, R., & Guttromson, R. (2018). Integrated Cyber/Physical Grid Resiliency Modeling. https://doi.org/10.2172/1482777 Publication ID: 60153
-
Sahakian, M., Verzi, S., Kouhestani, C., Birch, G., Stubbs, J., Woo, B.L., & Woo, B.L. (2018). Temporal Algorithms for Physical Security [Conference Poster]. https://www.osti.gov/biblio/1592359 Publication ID: 59683
-
Verzi, S., Rothganger, F., Parekh, O., Quach, T.-T., Miner, N., Vineyard, C., James, C., Aimone, J.B., & Aimone, J.B. (2018). Computing with spikes: The advantage of fine-grained timing. Neural Computation, 30(10), pp. 2660-2690. https://doi.org/10.1162/neco_a_01113 Publication ID: 63758
-
Naugle, A., Lakkaraju, K., Verzi, S., Swiler, L., Romero, V., Bernard, M., & Bernard, M. (2018). A Multi-tiered Complexity Metric [Conference Poster]. https://www.osti.gov/biblio/1570180 Publication ID: 63118
-
Verzi, S., Vineyard, C., Aimone, J.B., & Aimone, J.B. (2018). Neural-inspired Anomaly Detection [Conference Poster]. https://doi.org/10.1007/978-3-319-96661-8_21 Publication ID: 63164
-
Sahakian, M., Verzi, S., Kouhestani, C., Birch, G., Stubbs, J., Woo, B.L., & Woo, B.L. (2018). Temporal Algorithms for Physical Security [Conference Poster]. https://www.osti.gov/biblio/1573308 Publication ID: 63437
-
Vineyard, C., Verzi, S., Severa, W., Aimone, J.B., & Aimone, J.B. (2018). Spiking Neuron Implementations of Several Fundamental Machine Learning Algorithms [Conference Poster]. https://www.osti.gov/biblio/1498201 Publication ID: 60768
-
Verzi, S., Vineyard, C., Aimone, J.B., & Aimone, J.B. (2018). Neural-Inspired Anomaly Detection [Conference Poster]. Springer Proceedings in Complexity. https://doi.org/10.1007/978-3-319-96661-8_21 Publication ID: 62382
-
Hill, A.J., Donaldson, J., Rothganger, F., Vineyard, C., Follett, D.R., Follett, P.L., Smith, M., Verzi, S., Severa, W., Wang, F., Aimone, J.B., Naegle, J., James, C., & James, C. (2017). A spike-Timing neuromorphic architecture [Conference Poster]. 2017 IEEE International Conference on Rebooting Computing, ICRC 2017 – Proceedings. https://doi.org/10.1109/ICRC.2017.8123631 Publication ID: 58345
-
Vineyard, C., Verzi, S., & Verzi, S. (2017). A Case Study on Neural Inspired Dynamic Memory Management Strategies for High Performance Computing. https://doi.org/10.2172/1396076 Publication ID: 53208
-
Verzi, S., Dellana, R., Severa, W., Smith, M., Rothganger, F., James, C., Aimone, J.B., Vineyard, C., & Vineyard, C. (2017). Steep Deep Spiking Networks [Conference Poster]. https://www.osti.gov/biblio/1464724 Publication ID: 57912
-
Verzi, S., Vineyard, C., Vugrin, E., Galiardi, M., James, C., Aimone, J.B., & Aimone, J.B. (2017). Optimization-based computation with spiking neurons [Conference Poster]. Proceedings of the International Joint Conference on Neural Networks. https://doi.org/10.1109/IJCNN.2017.7966098 Publication ID: 54983
-
Sahakian, M., Verzi, S., Vineyard, C., Vugrin, E., James, C., Aimone, J.B., & Aimone, J.B. (2017). Optimization-based computation with spiking neurons [Conference Poster]. https://www.osti.gov/biblio/1457893 Publication ID: 56181
-
Vugrin, E., Trucano, T., Swiler, L., Finley, P., Flanagan, T., Naugle, A., Tsao, J., Verzi, S., & Verzi, S. (2017). Recommended Research Directions for Improving the Validation of Complex Systems Models. https://doi.org/10.2172/1367494 Publication ID: 54940
-
Jones, S., Outkin, A., Gearhart, J., Hobbs, J., Siirola, J.D., Phillips, C., Verzi, S., Tauritz, D.R., Mulder, S., Naugle, A., & Naugle, A. (2017). PLADD: Deterring Attacks on Cyber Systems and Moving Target Defense [Conference Poster]. https://www.osti.gov/biblio/1417561 Publication ID: 52843
-
Vineyard, C., Aimone, J.B., Verzi, S., Donaldson, J., Smith, M., Rothganger, F., Follet, D., James, C., Naegle, J., & Naegle, J. (2016). A neurally inspired spiking temporal processing unit computational architecture [Conference Poster]. https://www.osti.gov/biblio/1526843 Publication ID: 47696
-
Verzi, S., Vineyard, C., Vugrin, E., Sahakian, M., James, C., Aimone, J.B., & Aimone, J.B. (2016). Optimization-based computation with spiking neurons [Conference Poster]. https://doi.org/10.1109/IJCNN.2017.7966098 Publication ID: 48012
-
Verzi, S., Vineyard, C., Vugrin, E., Sahakian, M., James, C., Aimone, J.B., & Aimone, J.B. (2016). Optimization-based computation with spiking neurons [Conference Poster]. https://doi.org/10.1109/IJCNN.2017.7966098 Publication ID: 48013
-
Vineyard, C., Verzi, S., James, C., Aimone, J.B., & Aimone, J.B. (2016). Quantifying neural information content: A case study of the impact of hippocampal adult neurogenesis [Conference Poster]. Proceedings of the International Joint Conference on Neural Networks. https://www.scopus.com/inward/record.uri?partnerID=HzOxMe3b&scp=85007256437&origin=inward Publication ID: 45978
-
Stamber, K., Burnham, L., Adams, S., Jeffers, R., Verzi, S., & Verzi, S. (2016). Modeling the benefits (or detriments) of increased automation in electric power grid operations: Methodology and experiments [Conference Poster]. Electricity Journal. https://doi.org/10.1016/j.tej.2016.09.015 Publication ID: 51930
-
Stamber, K., Burnham, L., Adams, S., Jeffers, R., Verzi, S., & Verzi, S. (2016). Modeling the Benefits (or Detriments) of Increased Automation in Utility Operations [Presentation]. https://www.osti.gov/biblio/1390470 Publication ID: 52114
-
Outkin, A., Eames, B., Jones, S., Vugrin, E., Phillips, C., Walsh, S., Hobbs, J., Verzi, S., Heersink, B., & Heersink, B. (2016). A Framework for Analysis of Attacker-Defender Interaction in Cyber Systems [Conference Poster]. https://www.osti.gov/biblio/1392766 Publication ID: 52210
-
Burnham, L., Stamber, K., Jeffers, R., Adams, S., Verzi, S., Sahakian, M., Haass, M., Cauthen, K., & Cauthen, K. (2016). Improving Grid Resilience through Informed Decision-making (IGRID). https://doi.org/10.2172/1431483 Publication ID: 52545
-
Tsao, J., Trucano, T., Kleban, S., Naugle, A., Verzi, S., Swiler, L., Johnson, C.M., Smith, M., Flanagan, T., Vugrin, E., Gabert, K., Lave, M., Chen, W., DeLaurentis, D., Hubler, A., Oberkampf, B., & Oberkampf, B. (2016). Complex Systems Models and Their Applications: Towards a New Science of Verification, Validation & Uncertainty Quantification. https://doi.org/10.2172/1431458 Publication ID: 51944
-
Vineyard, C., Verzi, S., & Verzi, S. (2016). Overcoming the Static Learning Bottleneck – the Need for Adaptive Neural Learning [Conference Poster]. https://doi.org/10.1109/ICRC.2016.7738692 Publication ID: 50891
-
Outkin, A., Eames, B., Jones, T., Vugrin, E., Phillips, C., Walsh, S., Hobbs, J., Gearhart, J., Siirola, J.D., Verzi, S., Naugle, A., & Naugle, A. (2016). Stochastic Adversarial Modeling for Evaluating Trust in Systems and Effectiveness of Moving Target Defense [Presentation]. https://www.osti.gov/biblio/1530035 Publication ID: 50326
-
Outkin, A., Eames, B., Jones, T., Vugrin, E., Phillips, C., Walsh, S., Hobbs, J., Gearhart, J., Siirola, J.D., Verzi, S., Naugle, A., & Naugle, A. (2016). Stochastic Adversarial Modeling for Evaluating Trust in Systems and Effectiveness of Moving Target Defense [Presentation]. https://www.osti.gov/biblio/1530048 Publication ID: 50475
-
Verzi, S., Naugle, A., & Naugle, A. (2016). Model/Use Characteristics that Can Challenge Current VVUQ: Building Credible Models [Conference Poster]. https://www.osti.gov/biblio/1366869 Publication ID: 50715
-
Vineyard, C., Verzi, S., James, C., Aimone, J.B., & Aimone, J.B. (2016). Quantifying Neural Information Content: A Case Study of the Impact of Hippocampal Adult Neurogenesis [Conference Poster]. https://doi.org/10.1109/IJCNN.2016.7727884 Publication ID: 49412
-
Vineyard, C., Verzi, S., James, C., Aimone, J.B., & Aimone, J.B. (2016). Quantifying neural information content: a case study of the impact of hippocampal adult neurogenesis. https://doi.org/10.2172/1561002 Publication ID: 48705
-
Gearhart, J., Hobbs, J., Jones, S., Mulder, S., Naugle, A., Outkin, A., Phillips, C., Siirola, J.D., Tauritz, D.R., Verzi, S., & Verzi, S. (2016). A new scheduling problem motivated by moving-target cyberdefense [Conference Poster]. https://www.osti.gov/biblio/1364842 Publication ID: 49068
-
Naugle, A., Miner, N., Aamir, M., Jeffers, R., Verzi, S., Bernard, M., & Bernard, M. (2016). Simulating smoking behaviors based on cognition-determined, opinion-based system dynamics [Conference Poster]. Proceedings – Winter Simulation Conference. https://doi.org/10.1109/WSC.2015.7408545 Publication ID: 41829
-
Vineyard, C., Aimone, J.B., Bernard, M., Carlson, K.D., Chance, F., Forsythe, J., James, C., Rothganger, F., Severa, W., Speed, A., Verzi, S., Warrender, C., Wagner, J., Miller, L., & Miller, L. (2015). Neural Computing at Sandia National Laboratories [Conference Poster]. https://www.osti.gov/biblio/1335732 Publication ID: 41869
-
Vineyard, C., Verzi, S., James, C., Aimone, J.B., Heileman, G.L., & Heileman, G.L. (2015). Repeated play of the SVM game as a means of adaptive classification [Conference Poster]. Proceedings of the International Joint Conference on Neural Networks. https://www.scopus.com/inward/record.uri?partnerID=HzOxMe3b&scp=84951142808&origin=inward Publication ID: 43179
-
Jones, S., Outkin, A., Gearhart, J., Hobbs, J., Siirola, J.D., Phillips, C., Verzi, S., Tauritz, D.R., Mulder, S., Naugle, A., & Naugle, A. (2015). Evaluating Moving Target Defense with PLADD. https://doi.org/10.2172/1222986 Publication ID: 45812
-
Vineyard, C., Verzi, S., James, C., Aimone, J.B., Heileman, G., & Heileman, G. (2015). Repeated Play of the SVM Game as a Means of Adaptive Classification [Conference Poster]. https://doi.org/10.1109/IJCNN.2015.7280729 Publication ID: 44877
-
Vineyard, C., Verzi, S., James, C., Aimone, J.B., Heileman, G., & Heileman, G. (2015). MapReduce SVM Game [Conference Poster]. https://www.osti.gov/biblio/1256556 Publication ID: 43816
-
Finley, P., Vugrin, E., Verzi, S., Turnquist, M., Griffin, A., Ricci, K., Wyte-Lake, T., & Wyte-Lake, T. (2015). Modeling Hospital Evacuations for Infrastructure Disruptions [Conference Poster]. https://www.osti.gov/biblio/1331571 Publication ID: 44088
-
Moffett, M., Farnbach Pearson, A., Verzi, S., Kleban, S., Malczynski, L., McDermott, G., Larson, R., & Larson, R. (2015). Modeling Future Health Care Workforce Adequacy to Inform Policy [Conference Poster]. https://www.osti.gov/biblio/1530991 Publication ID: 43258
-
Vugrin, E., Rostron, B.L., Verzi, S., Brodsky, N., Brown, T.J., Choiniere, C.J., Coleman, B.N., Paredes, A., Apelberg, B.J., & Apelberg, B.J. (2015). Modeling the potential effects of new tobacco products and policies: A dynamic population model for multiple product use and harm. PLoS ONE, 10(3). https://doi.org/10.1371/journal.pone.0121008 Publication ID: 38308
-
Brown, T., Vugrin, E., Tidwell, V., Verzi, S., Moore, T., Finley, P., Beyeler, W., Corbet, T., & Corbet, T. (2015). Complex System Analytics: presentations to External Advisory Panel [Conference Poster]. https://www.osti.gov/biblio/1247089 Publication ID: 42410
-
Verzi, S., Vugrin, E., Brodsky, N., Malczynski, L., Kleban, S., & Kleban, S. (2015). Dynamic Population Health Modeling [Presentation]. https://www.osti.gov/biblio/1513983 Publication ID: 42573
-
Vugrin, E., Verzi, S., Forden, G., Aamir, M., Lamb, C., Outkin, A., & Outkin, A. (2015). Measuring & Influencing Resilience of Adapting Flow Networks [Presentation]. https://www.osti.gov/biblio/1244482 Publication ID: 42580
-
Vugrin, E.D., Verzi, S., Finley, P., Turnquist, M.A., Griffin, A.R., Ricci, K.A., Wyte-Lake, T., & Wyte-Lake, T. (2015). Modeling Evacuation of a Hospital without Electric Power. Prehospital and Disaster Medicine, 30(3), pp. 279-287. https://www.scopus.com/inward/record.uri?partnerID=HzOxMe3b&scp=84930275101&origin=inward Publication ID: 41087
-
Vugrin, E., Verzi, S., Finley, P., Turnquist, M., Wyte-Lake, T., Griffin, A., Ricci, K., Plotinsky, R., & Plotinsky, R. (2015). Resource Requirements Planning for Hospitals Treating Serious Infectious Disease Cases. https://doi.org/10.2172/1171661 Publication ID: 42234
-
Naugle, A., Miner, N., Aamir, M., Jeffers, R., Verzi, S., Bernard, M., & Bernard, M. (2015). Simulating Smoking Behaviors Based on Cognition-Determined Communication of Opinions [Conference Poster]. https://www.osti.gov/biblio/1504118 Publication ID: 41361
-
Backus, G.A., Naugle, A., Bernard, M., Aamir, M., Glickman, M., Jeffers, R., Silva, A., Trumbo, D., Verzi, S., & Verzi, S. (2015). Simulating Behavioral Responses in International Security Settings [Conference Poster]. https://www.osti.gov/biblio/1244850 Publication ID: 42661
-
Beyeler, W., Vugrin, E., Forden, G., Aamir, M., Verzi, S., Outkin, A., & Outkin, A. (2015). Resilience of Adapting Networks: Results from a Stylized Infrastructure Model. https://www.osti.gov/biblio/1427206 Publication ID: 42673
-
Verzi, S., Aimone, J.B., & Aimone, J.B. (2014). Quantification of Neural Computation [Conference Poster]. https://www.osti.gov/biblio/1315443 Publication ID: 39580
-
Naugle, A., Miner, N., Aamir, M., Jeffers, R., Verzi, S., Bernard, M., & Bernard, M. (2014). Simulating Smoking Behaviors Based on Cognition-Determined Opinion Dynamics [Conference Poster]. https://doi.org/10.1109/WSC.2015.7408545 Publication ID: 39124
-
Aimone, J.B., Bernard, M., Vineyard, C., Verzi, S., & Verzi, S. (2014). Using High Performance Computing to Examine the Processes of Neurogenesis Underlying Pattern Separation and Completion of Episodic Information. https://doi.org/10.2172/1162373 Publication ID: 39168
-
Brodsky, N., Brown, T., Verzi, S., Vugrin, E., Finley, P., & Finley, P. (2014). Tobacco-Control Regulatory Policy Modeling and Analysis [Presentation]. https://www.osti.gov/biblio/1706361 Publication ID: 40478
-
Vugrin, E., Verzi, S., Brodsky, N., & Brodsky, N. (2013). Population Structure Modeling to Evaluate Substitution and Dual Use of Tobacco Products in Response to Changing Policies [Conference]. https://www.osti.gov/biblio/1115323 Publication ID: 34816
-
Verzi, S., Bernard, M., Aimone, J.B., & Aimone, J.B. (2013). Adult Neurogenesis: Implications on Human And Computational Decision Making [Conference]. https://www.osti.gov/biblio/1145214 Publication ID: 32435
-
Brown, T., Brodsky, N., Verzi, S., & Verzi, S. (2012). Modeling Population Dynamics [Conference]. https://www.osti.gov/biblio/1063540 Publication ID: 26465
-
Vineyard, C., Verzi, S., Avina, G., & Avina, G. (2012). Copy of Copy of Neurogenesis in a High Resolution Dentate Gyrus Model [Conference]. https://www.osti.gov/biblio/1141197 Publication ID: 30794
-
Vineyard, C., Verzi, S., Avina, G., & Avina, G. (2012). Copy of Copy of Neurogenesis in a High Resolution Dentate Gyrus Model [Conference]. https://www.osti.gov/biblio/1141250 Publication ID: 30795
-
Speed, A., Vineyard, C., Collard, J., Verzi, S., & Verzi, S. (2012). Diffusion among cognitively complex agents : final report. https://doi.org/10.2172/1055582 Publication ID: 30258
-
Carter, C., Verzi, S., Hensley, C., & Hensley, C. (2012). Space Based Solar Power [Presentation]. https://www.osti.gov/biblio/1650318 Publication ID: 28589
-
Verzi, S., Bernard, M., Taylor, S., Dubicka, I., & Dubicka, I. (2012). Associative-ART: A Multi-modal Network Architecture for Knowledge Discovery. Proposed for publication in Security Informatics., 1(1). https://doi.org/10.1186/2190-8532-1-20 Publication ID: 28138
-
Verzi, S. (2012). NEUROCOMPUTATION BY A NEURAL CHIP-FIRING GAME [Conference]. https://www.osti.gov/biblio/1073374 Publication ID: 28345
-
Vineyard, C.M., Lakkaraju, K., Collard, J., Verzi, S., & Verzi, S. (2012). The impact of attitude resolve on population wide attitude change [Conference]. Lecture Notes in Computer Science (including subseries Lecture Notes in Artificial Intelligence and Lecture Notes in Bioinformatics). https://www.scopus.com/inward/record.uri?partnerID=HzOxMe3b&scp=84859150989&origin=inward Publication ID: 25400
-
Verzi, S. (2012). A Neural Chip-Firing Game as a Model of Neurocomputation [Conference]. https://www.osti.gov/biblio/1078716 Publication ID: 26689
-
Vineyard, C., Verzi, S., Taylor, S., Dubicka, I., Bernard, M., & Bernard, M. (2011). Augmented cognition tool for rapid military decision making. https://doi.org/10.2172/1029756 Publication ID: 24737
-
Naugle, A., Bernard, M., Backus, G.A., Glickman, M., Verzi, S., & Verzi, S. (2011). Using a hybrid cognitive-system dynamics model to anticipate the influence of events and actions on human behaviors [Conference]. https://www.osti.gov/biblio/1290129 Publication ID: 23681
-
Finley, P., Linebarger, J., Outkin, A., Verzi, S., Brodsky, N., Glass, R., & Glass, R. (2011). Extending Opinion Dynamics to Model Public Health Problems and the Evaluation of Policy Interventions [Conference]. https://www.osti.gov/biblio/1106717 Publication ID: 22704
-
Verzi, S., Bernard, M., Taylor, S., Dubicka, I., McClain, J., & McClain, J. (2011). A Neurophysiologically Inspired Hippocampus Based Associative-ART Artificial Neural Network Architecture [Conference]. https://www.osti.gov/biblio/1106201 Publication ID: 22716
-
Glass, R., Zagonel, A., Ames, A., Brown, T., Beyeler, W., Finley, P., Linebarger, J., Brodsky, N., Verzi, S., Outkin, A., & Outkin, A. (2011). Complex Adaptive Systems of Systems (CASoS) Engineering: Mapping Aspirations to Problem Solutions [Conference]. https://www.osti.gov/biblio/1106542 Publication ID: 22783
-
Bernard, M., Verzi, S., Taylor, S., Dubicka, I., McClain, J., Vineyard, C., & Vineyard, C. (2011). Augmented Cognition Tool for Rapid Military Decision Making (ACORD) [Presentation]. https://www.osti.gov/biblio/1671500 Publication ID: 22272
-
Finley, P., Linebarger, J., Outkin, A., Verzi, S., Brodsky, N., Cannon, D., Glass, R., & Glass, R. (2011). Extending Opinion Dynamics to Model Public Health Problems and the Evaluation of Policy Interventions [Conference]. https://www.osti.gov/biblio/1120573 Publication ID: 22296
-
Bernard, M., Backus, G.A., Glickman, M., Verzi, S., & Verzi, S. (2011). Using a Hybrid Cognitive-System Dynamics Model to Anticipate the Influence of Events and Actions on Human Behaviors [Conference]. https://www.osti.gov/biblio/1109155 Publication ID: 21852
-
Bernard, M., Dubicka, I., McClain, J., Taylor, S., Verzi, S., Vineyard, C., & Vineyard, C. (2011). A Neurophysiologically Inspired Hippocampus Based Associative-ARTMAP Artificial Neural Network Architecture [Conference]. https://www.osti.gov/biblio/1109437 Publication ID: 21591
-
Verzi, S., Rohrer, B., Xavier, P., Rothganger, F., & Rothganger, F. (2011). Neurons to Algorithms [Presentation]. https://www.osti.gov/biblio/1671628 Publication ID: 21738
-
Rothganger, F., Rohrer, B., Verzi, S., Xavier, P., & Xavier, P. (2010). Modeling cortical circuits. https://doi.org/10.2172/1008124 Publication ID: 19889
-
Verzi, S., Wagner, J., Warrender, C., & Warrender, C. (2010). Optical holography as an analogue for a neural re-use mechanism: Comment on Anderson (2010). Behavioral and Brain Sciences, 33(04). https://doi.org/10.1017/S0140525X10001214 Publication ID: 18645
-
Verzi, S., Bernard, M., Quach, T.-T., Taylor, S., & Taylor, S. (2010). A Cortical-Hippocampal Neural Architecture for Episodic Memory with Information Theoretic Model Analysis [Conference]. https://www.osti.gov/biblio/1123559 Publication ID: 18512
-
Speed, A., Verzi, S., Wagner, J., Warrender, C., & Warrender, C. (2010). Proposed comment on Anderson (2010) Neural re-use as a fundamental organizational principle of the brain. Behavioral Brain Science. https://www.osti.gov/biblio/1124264 Publication ID: 17799
-
Bernard, M., Morrow, J., Taylor, S., Verzi, S., Vineyard, C., & Vineyard, C. (2009). Modeling aspects of human memory for scientific study. https://doi.org/10.2172/986595 Publication ID: 16784
-
Taylor, S., Bernard, M., Vineyard, C., Verzi, S., Morrow, J., & Morrow, J. (2009). Memory in Silico: Building a Neuromimetic Episodic Cognitive Model [Conference]. https://www.osti.gov/biblio/1142499 Publication ID: 16064
-
Djordjevich Reyna, D., Xavier, P., Bernard, M., Whetzel, J., Glickman, M., Verzi, S., & Verzi, S. (2008). Preparing for the Aftermath: Using Emotional Agents in Game-Based Training for Disaster Response [Conference]. https://www.osti.gov/biblio/1142849 Publication ID: 14475
-
Chew, P., Bader, B., Verzi, S., & Verzi, S. (2008). Can computational linguistics improve information retrieval?. Proposed for publication in Computational Linguistics.. https://www.osti.gov/biblio/948222 Publication ID: 14599
-
Dunlavy, D., Basilico, J.D., Verzi, S., Bauer, T., & Bauer, T. (2008). Yucca Mountain licensing support network archive assistant. https://doi.org/10.2172/932885 Publication ID: 12927
-
Bernard, M., Glickman, M., Hart, D., Xavier, P., Verzi, S., Wolfenbarger, P., & Wolfenbarger, P. (2007). Simulating human behavior for national security human interactions. https://doi.org/10.2172/900422 Publication ID: 8609
-
Verzi, S., Chew, P., Bauer, T., McClain, J., & McClain, J. (2006). Cross-Language Ideology Detection Using Linguistics-Based Knowledge Extraction [Presentation]. https://www.osti.gov/biblio/1725704 Publication ID: 8103
-
Chew, P., Verzi, S., McClain, J., Bauer, T., & Bauer, T. (2006). Evaluation of the Bible as a Resource for Cross-Language Information Retrieval [Conference]. https://www.osti.gov/biblio/1264239 Publication ID: 7477
-
Verzi, S., Chew, P., & Chew, P. (2006). Database of on-line Bible texts in 6 langauges as well as web-spidered web pages [Presentation]. https://www.osti.gov/biblio/1729825 Publication ID: 7381
-
Chew, P., Bauer, T., Verzi, S., McClain, J., & McClain, J. (2006). Evaluation of the Bible as a resource for cross-language information retrieval [Conference]. https://www.osti.gov/biblio/943892 Publication ID: 6872
Showing 10 of 135 publications.
Patents & Trademarks
- (App #12/364,753; Patent #US 8166051 B1), Bauer, T.L., Benz, Z.O., & Verzi, S.J. Computation of term dominance computation in text documents, Filed: 02/03/2009, Granted: 06/24/2012.
- (App #15/837,326; Pub. No.: US 2019/0180169 A1), Verzi, S.J., Vineyard, C.M., Miner, N.E., & Aimone, J.B. Optimization computation with spiking neurons, Filed: 12/11/2017, Published: 06/13/2019.
- (App #16/013,810) Devices and methods for increasing the speed or power efficiency of a computer when performing machine learning using spiking neural networks,,Vineyard, C.M., Aimone, J.B. Severa, W.M., & Verzi, S.J. Filed: 06/20/2018.
- Verzi, S.J., Vineyard, C.M., & Aimone, J.B. Anomaly detection with spiking neural networks (App #16/436,744), Filed 06/10/2019.